SPOTLIGHT
By Anette Granly Koch, Ph.D., Scientific Manager, Danish Meat Research Institute (DMRI), Danish Technological Institute (DTI), and Gry Carl Terrell, M.Sc., Business Manager, DMRI, DTI
Predictive Models for Ensuring the Safety and Quality of Meat and Meat Alternatives
As more plant-based meat alternatives enter the market, the need for predictive models to predict the safety and shelf life of these products grows
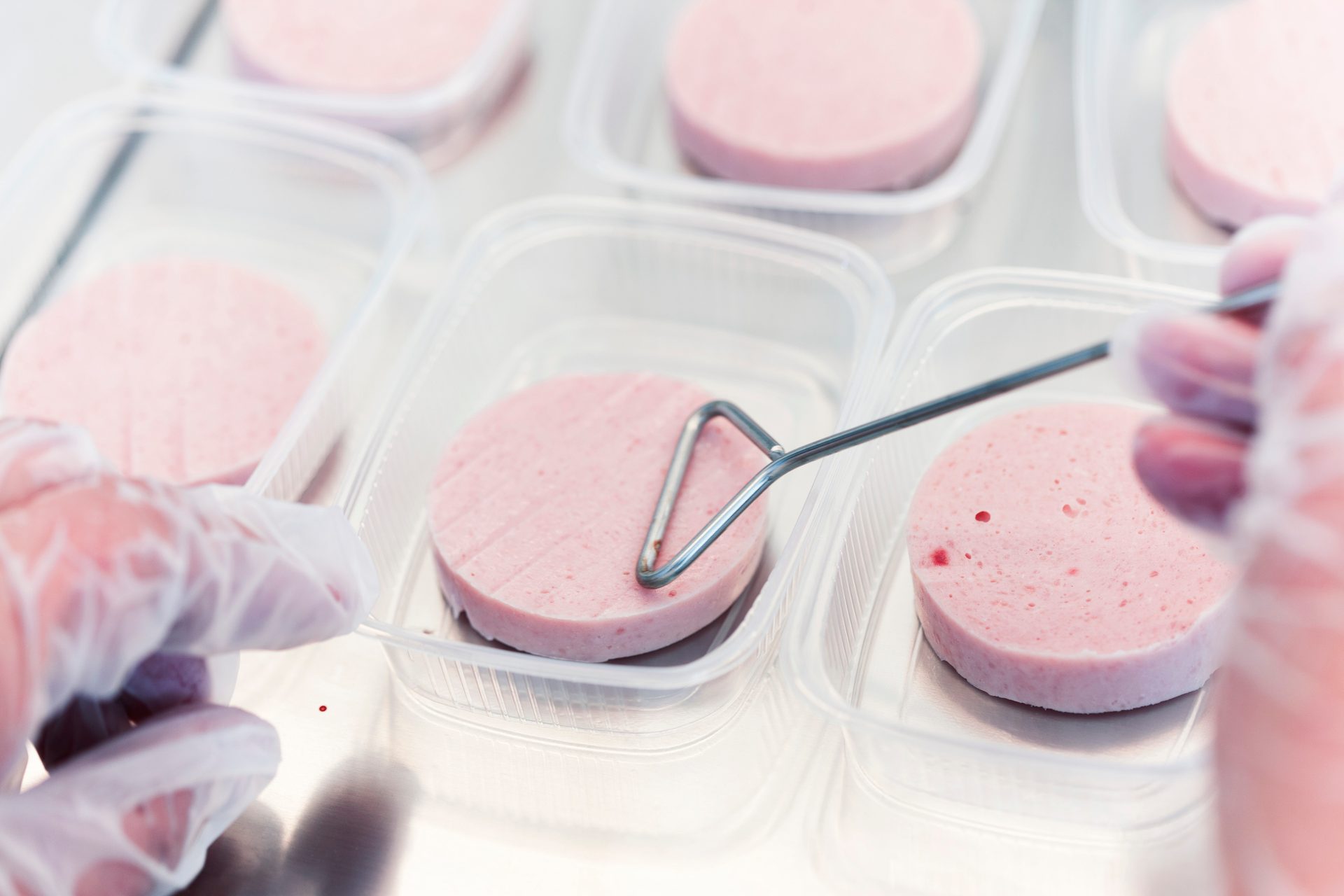
Image credit: Danish Technological Institutes
SCROLL DOWN
A predictive model is a mathematical framework or algorithm used to forecast future outcomes based on historical data. These models analyze patterns and relationships within the data to make informed predictions about unknown or future events. Mathematical models can predict how bacteria will behave under different environmental conditions such as temperature, pH, and water activity. They have become indispensable tools for the food industry, used widely for predicting both the safety and shelf life of foods.
Predictive models allow for the simulation of various storage and handling scenarios, as well as preservation techniques to assess their impact on food safety and shelf life. This helps in decision-making related to effective preservation strategies, packaging, distribution, and storage conditions. The models can also serve as documentation for customers, regulatory entities, and third-party auditors.
DMRI Predict is a collection of open-access predictive models that can be used to assess food safety and spoilage (both microbiological and sensory) of meat and meat products. The models have been developed in collaboration with the meat industry and are based on a large number of shelf life and challenge tests conducted with meat.
With around 2,000 registered users from Denmark and abroad, the Danish Meat Research Institute's (DMRI's) DMRI Predict1 (Figure 1) plays an important role in ensuring that meat and meat products worldwide are produced in a safe manner and with a reliable shelf life determination. DMRI Predict is open for all and free of charge to use.
FIGURE 1. DMR Predict online welcome screen (image courtesy of the DMRI)
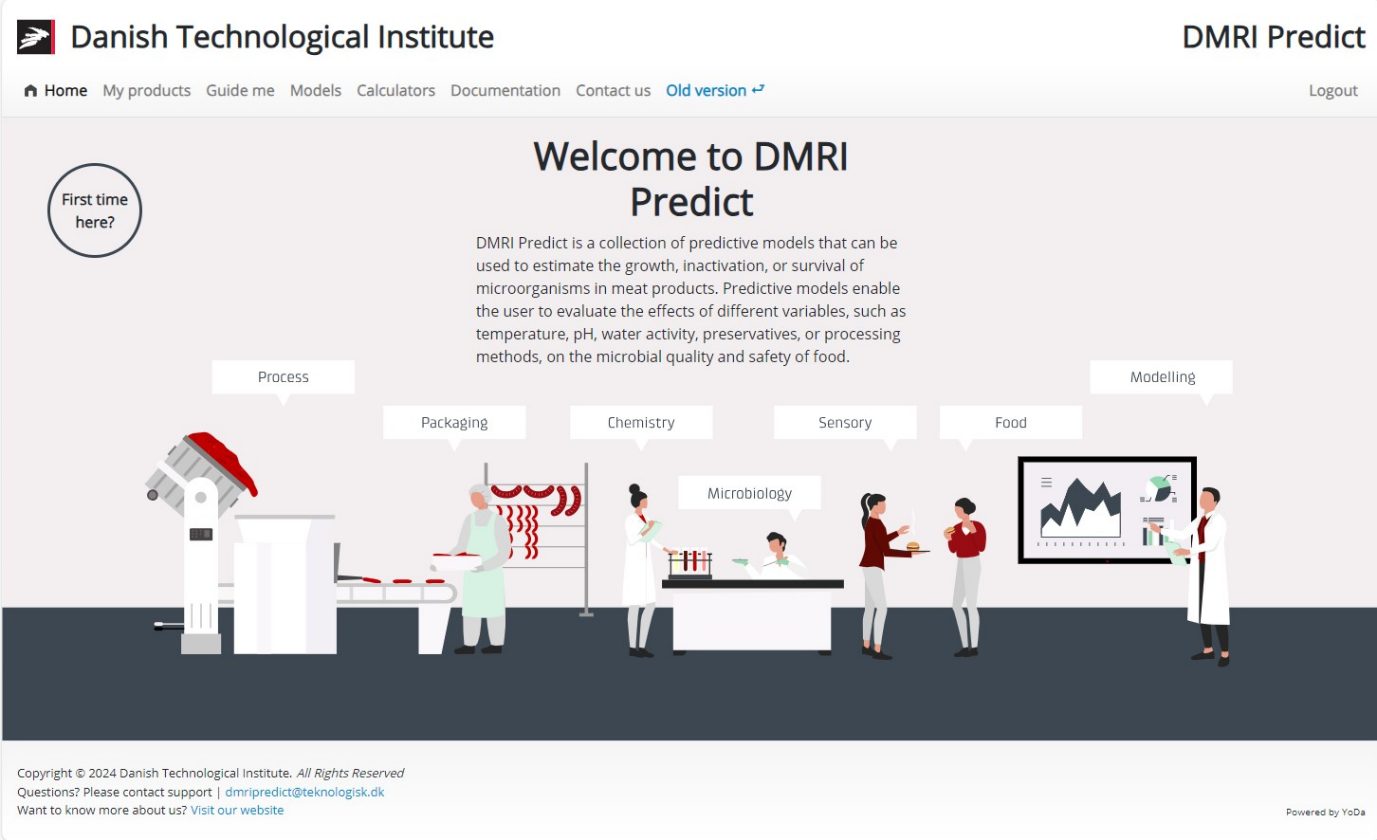
Work of Continuous Improvement
Recently, DMRI Predict was given a major makeover, with new features added and improvements made to the user experience. The DMRI continues to add content in the form of new models or improvements to existing models.
There is a growing industry for meat alternatives—i.e., plant-based and hybrid products. As more and more of these meat alternatives enter the market, the need for predictive models that can be used to predict the safety and shelf life of these products grows. In recognition of this need, the DMRI is looking at several of its models to see if they can be used for meat alternatives and, if possible, make the adjustments needed.
The average user is often not aware of the extensive work that lies behind developing predictive models, but the following two examples will give insight into the considerations and work that it entails.
The shelf life models within DMRI Predict are usually based on microbial analysis of meat collected from different companies in multiple countries, after which the natural spoilage flora is analyzed over time, thus modeling "real-world" conditions and all the variation that this represents. The example Leuconostoc model below describes how a shelf-life model based on the growth of a specific group of bacteria is developed and validated.
The second example, the Bacillus cereus model, demonstrates the work that goes into developing the DMRI Predict safety models, where planning appropriate challenge studies is crucial.
“Introduction of a predictive growth model for gas-producing bacteria can help prevent spoilage and thereby reduce food waste, as microorganisms show response to environmental factors that can be predicted by a mathematical model, such as the newly developed Leuconostoc model for MA-packaged deli meats.”

Shelf Life Models
Applying vacuum or modified atmosphere (MA) to food packages serves as a method for extending the shelf life of food products, as the surrounding atmosphere in the packaging can become a less favorable environment for aerobic spoilage microorganisms.2,3 In contrast, removing oxygen from packaged food (i.e., applying vacuum) creates a favorable environment for other spoilage microorganisms like lactic acid bacteria (LAB). Compared to aerobic spoilage bacteria, spoilage LAB produce their typical sensory changes (such as souring, gas formation, and/or slime formation) later, at the stationary phase.4
LAB can grow under anaerobic conditions (i.e., absence of oxygen), a pH of around 6, and refrigerated temperatures; therefore, psychrotrophic LAB like Leuconostoc spp. are selected for when oxygen is removed from food products.5–8 Specifically, Leuconostoc carnosum and Leuconostoc mesenteroides are associated with spoilage of deli meat products, which are packaged with MA or vacuum.6,9 In addition, L. mesenteroides has also been observed as a significant spoiler in plant-based products. Consequently, massive quantities of deli food products are discarded annually due to unwanted gas production caused by spoilage by microorganisms (usually LAB, e.g., Leuconostoc spp.). This spoilage may result in blown packages (Figure 2) and reduced shelf life.
Introduction of a predictive growth model for gas-producing bacteria can help prevent spoilage and thereby reduce food waste, as microorganisms show response to environmental factors (e.g., preservatives) that can be predicted by a mathematical model, such as the newly developed Leuconostoc model for MA-packaged deli meats.
FIGURE 2. Gas production in vacuum-packed deli meat inoculated with a Leuconostoc cocktail (mix of 11 strains) (image courtesy of the DMRI)
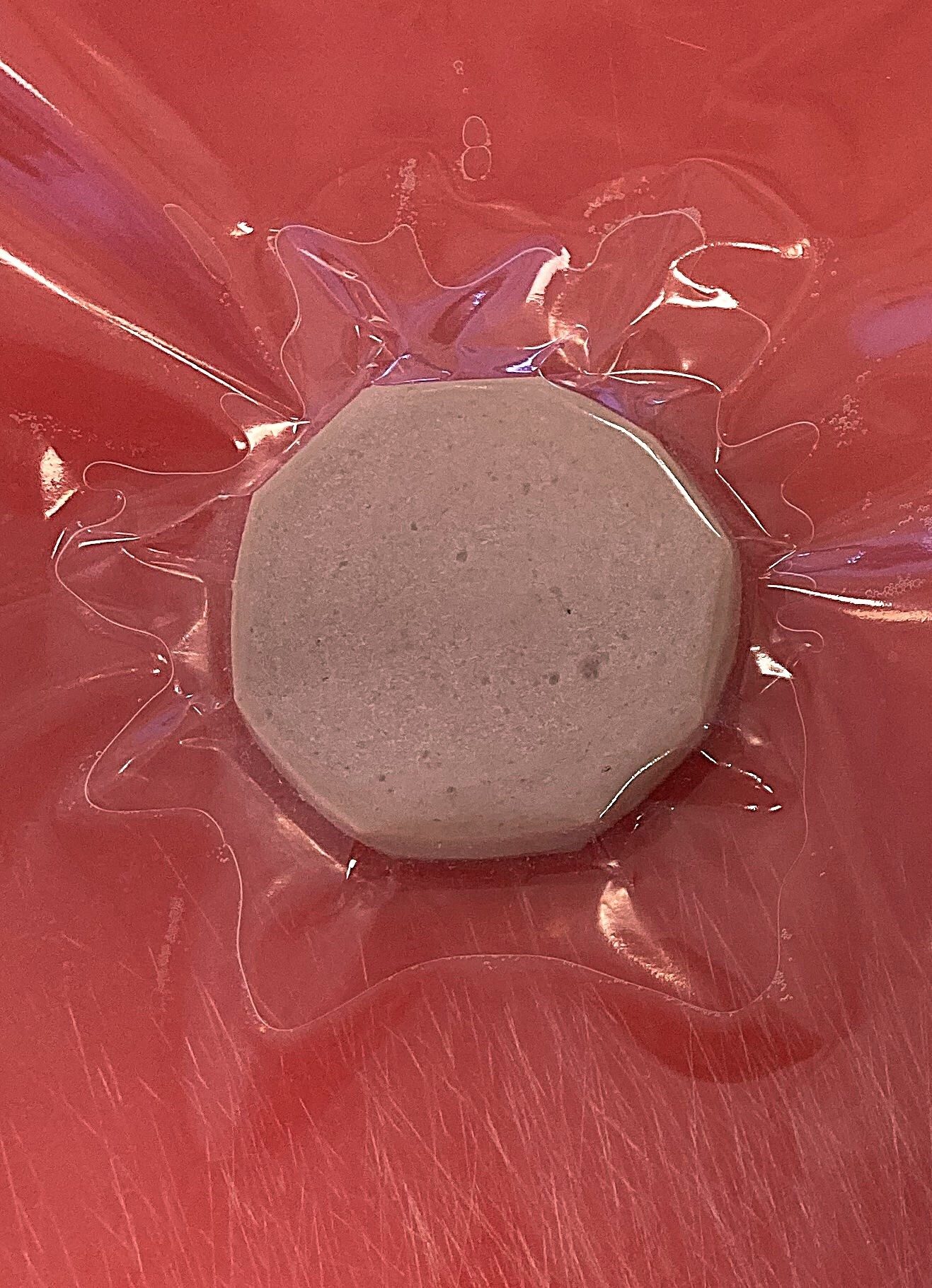
Model Development
Blown packs of deli meat were obtained from Danish retailers, and dominating gas producers were identified using 16S sequencing and whole genome sequencing (WGS) to identify differences in Leuconostoc carnosum and Leuconostoc mesenteroides strains isolated from different producers. Eleven strains were chosen for the development of the predictive growth model. Model products of deli meat and plant-based sausages were manufactured at the in-house pilot plant facilities at the DMRI, sliced under sterile conditions, inoculated with the 11-strain Leuconostoc cocktail, MA packaged (30 percent CO2/70 percent N2), and stored at temperatures between 3 °C and 8 °C. Subsequently, data for the growth model was collected by analyzing growth on the inoculated deli meat slices produced with varying combinations of pH, NaCl, Na-nitrite, Na-lactate, and Na-acetate (see Table 1 for an overview).
TABLE 1. Overview of Parameters and Ranges Included in the Leuconostoc Model
Based on the collected data, a model for growth of Leuconostoc was developed. Maximum growth rates (µmax) for the model were estimated using ComBase online Dynamic Modeling Fit (DMFit).10 The Baranyi model with no lag phase was employed, since this type of model applied to most samples.
The Leuconostoc model is a linear regression model fitted using Python. During the development of the model, 27 maximum growth rates were used for internal evaluation of the model's predictability of µmax [log10 (CFU/g/h)]. The final model accounts for the effect of temperature (°C), pH, salt, lactate (percent), and acetate (percent) in the water phase, as well as a combination of pH and each of the organic acids affecting the growth rate of Leuconostoc spp.
Challenge tests showed that the addition of Na-nitrite had no significant effect on the growth of the studied Leuconostoc cocktail. This observation is consistent with Ji et al.,11 who observed no effect of Na-nitrite on the growth rate of Leuconostoc spp. However, as Na-nitrite is a commonly used preservative in processed meat, Na-nitrite (ppm) was included in the model.
Model Validation
The model was validated against data from previous projects and from new shelf life studies on other types of meat products (baloney and ham), where the spoilage was caused by Leuconostoc spp. In all cases, the meat was inoculated with the Leuconostoc cocktail. There was a good fit between observed and predicted values, as shown in Figure 3.
Validation of the model (n = 52) showed that it was acceptable with a bias factor (Bf) of 0.96 and an accuracy factor (Af) of 1.18.
FIGURE 3. Example of growth observed (dots) vs. growth predicted (line) by the DMRI Leuconostoc model during the validation step
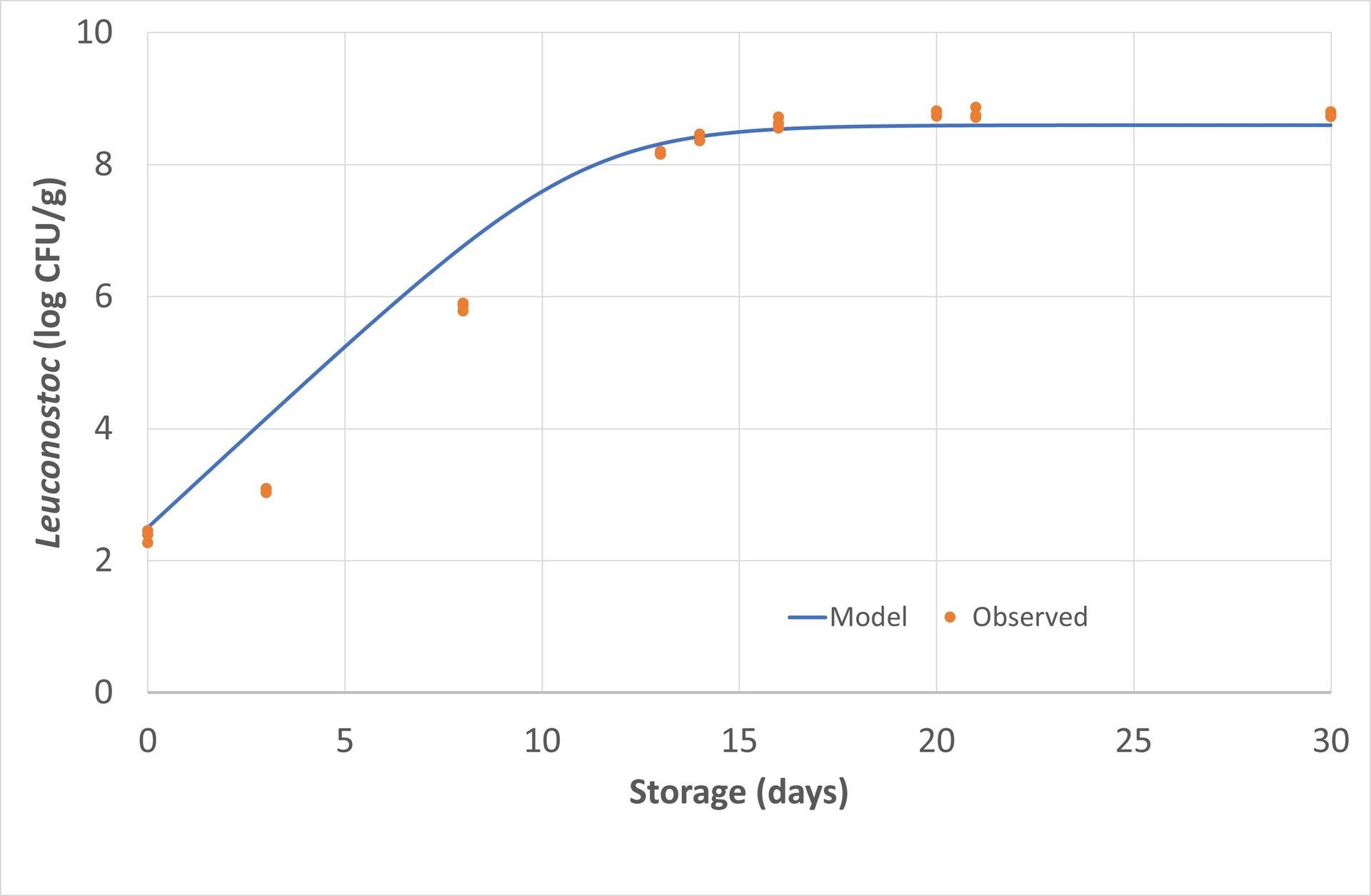
The model was recently validated against plant-based sausages. The sausage recipes contained 1 percent NaCl and either 0 percent or 2.8 percent Na-lactate and were inoculated with the same cocktail of Leuconostoc strains as used for developing the model for meat. The sausages were stored at two temperatures, 3 °C and 7 °C, respectively. As an example, Figure 4 compares the growth observed in the inoculated plant-based sausages (added Na-lactate) with the growth predicted by the meat model at 3 °C and 7 °C, respectively.
Similar good fits between observed and predicted values for the rest of the dataset lead to the conclusion that the risk of blown packages due to CO2 production by Leuconostoc is close to the same for plant-based sausages as for meat sausages. Therefore, the existing meat model can be used as an acceptable tool to predict Leuconostoc growth in plant-based deli products. However, additional testing would be beneficial to build an even more solid base for the model.
FIGURE 4. Example of growth observed (dots) in plant-based sausages with added 1 percent NaCl and 2.8 percent Na-lactate vs. growth predicted (lines) by the DMRI Leuconostoc model during the validation against plant-based deli-meat. Note: Blue dots and lines are at 3 °C, and orange dots and lines are at 7 °C.
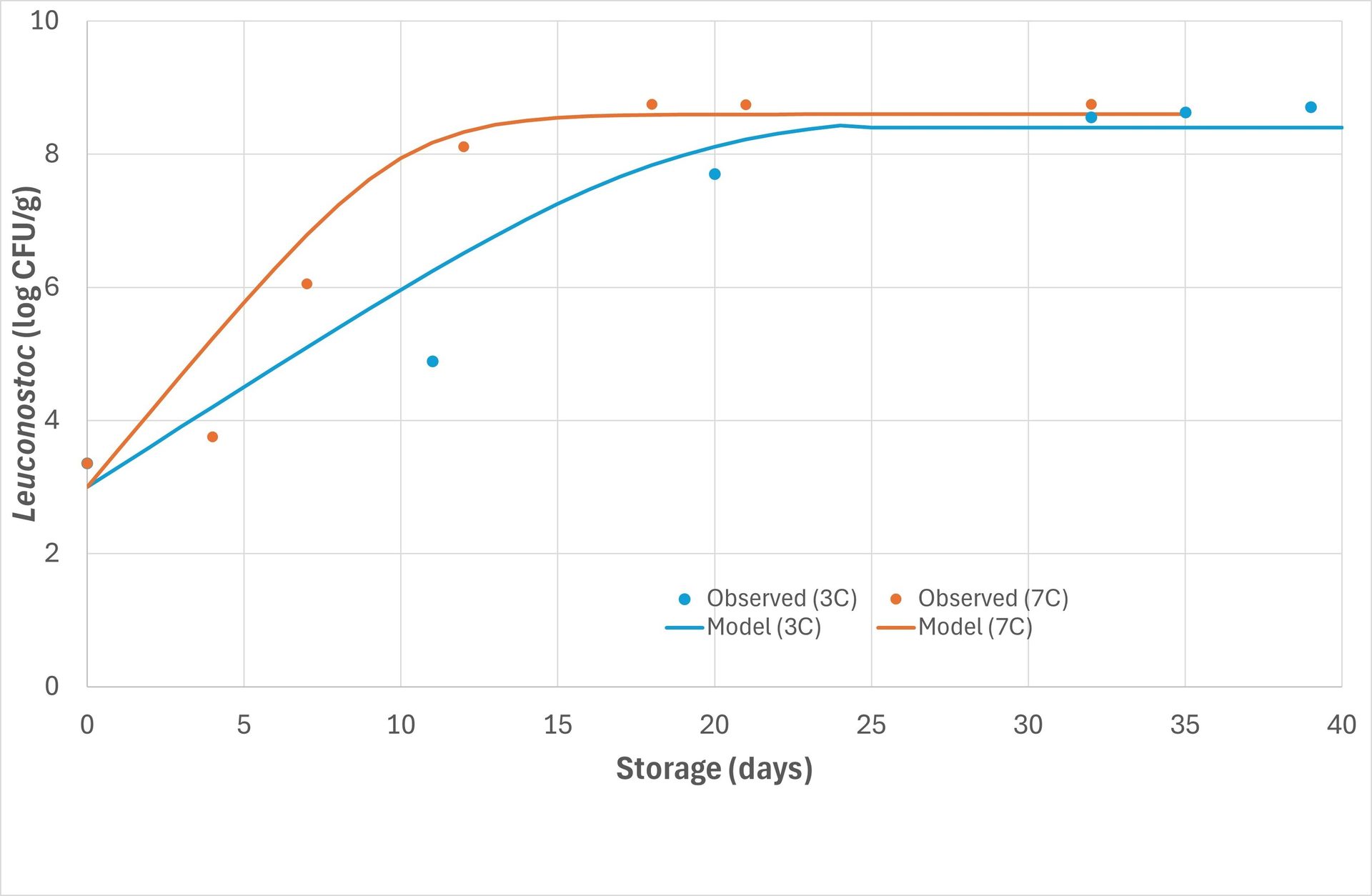
Safety Models
Bacillus cereus can cause food poisoning via two types of illness caused by two distinct metabolites, or toxins. A diarrheal illness is caused by a large-molecular-weight protein, and a vomiting (emetic) illness is associated with cereulide, an ionophoric, low molecular-weight dodecadepsipeptide that is pH-stable and heat- and protease- resistant. The non-antigenic peptide is stable after heating at 121 °C for 30 minutes, cooling at 4 °C for 60 days, and at a pH range of 2–11.12
B. cereus is widely distributed and occurs in many foods. It is often found in spices and dried ingredients, where it survives in spore form. In heat-treated foods, the Bacillus spore must be prevented from germinating and starting growth. B. cereus is a facultative, anaerobic, spore-forming bacteria, with great variation in growth characteristics (Figure 5). Some B. cereus strains can grow at temperatures as low as 4 °C, although growth is very restricted, while other B. cereus strains do not grow at temperatures below 10 °C. In an ongoing project, the DMRI is developing a mathematical model that can predict the growth of B. cereus in heat-treated deli meat products that are stored vacuum packed or MA packaged (30 percent CO2/70 percent N2) at refrigeration.
FIGURE 5. Bacillus cereus grown on agar plate (image courtesy of the DMRI)
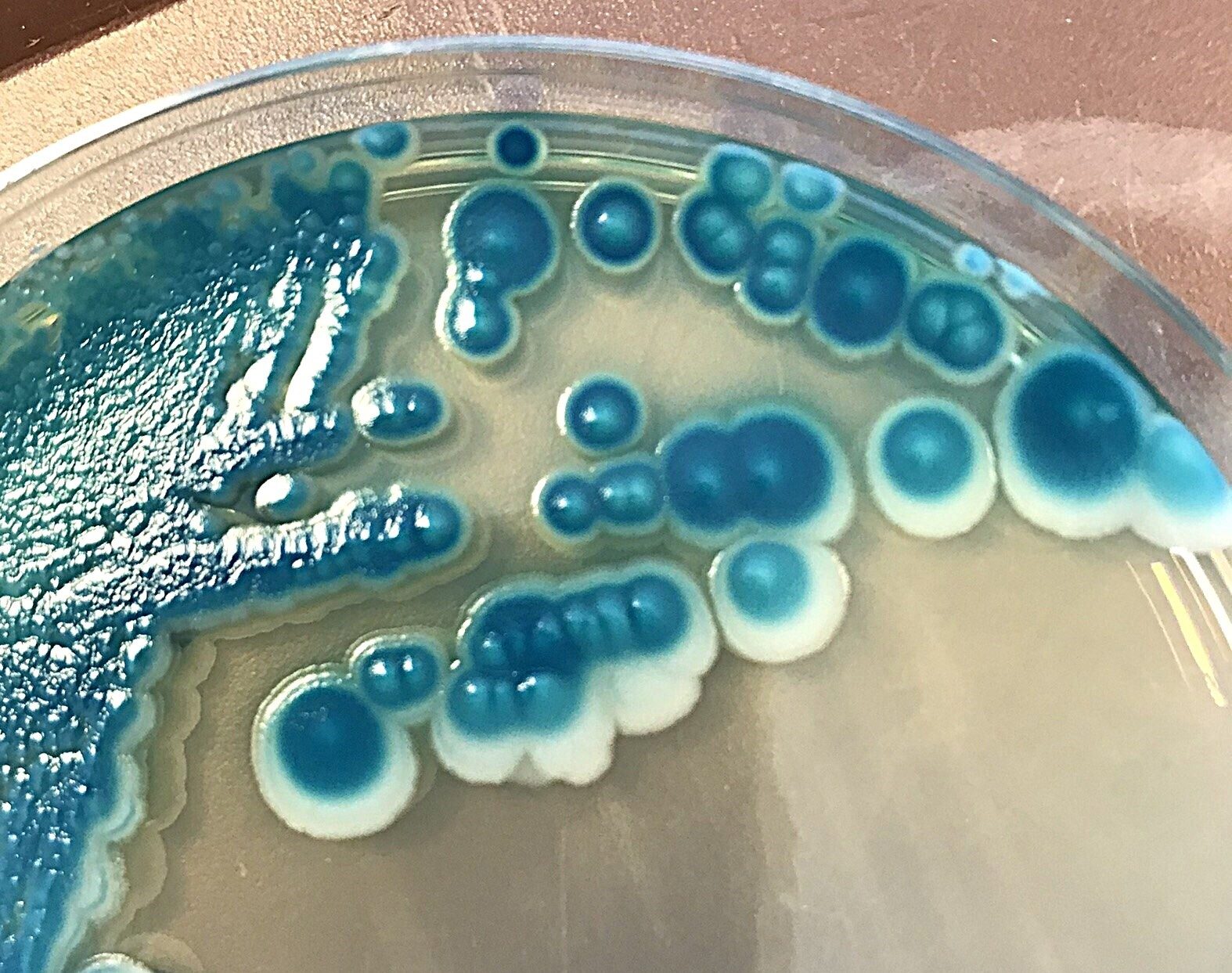
Oxygen Residue and Packaging Integrity
For a B. cereus spore to germinate, there must be some oxygen present in the package. The oxygen concentration in a package depends on how much oxygen is left after the packaging process and how airtight the packaging is (i.e., how well it prevents oxygen from diffusing into the package from the surrounding air). Assuming that the seal is tight, the amount of oxygen that enters the package depends on the oxygen transmission rate (OTR) of the packaging material.
Previous studies have shown that if inoculated cold cuts [pH 6.2, 2.2 percent salt in aqueous phase (SAP)] were MA packaged (20 percent CO2/80 percent N2) in a very airtight package (OTR 1.3 ml/m2/24 hours), there was no growth of three different B. cereus strains at 8 °C for four weeks of storage. However, if the packaging was less airtight (OTR: 40 ml/m2/24 hours), then two of the three strains were able to grow in the product. If the inoculated product was packaged with a residual oxygen concentration of 2 percent, then all three strains were able to grow in the product.13
Vacuum Packaging vs. Modified Atmosphere Packaging
The DMRI's recent experiments have shown that MA packing in 30 percent CO2/70 percent N2 (0.03 percent residual oxygen) provides a significantly better inhibition of B. cereus than vacuum packing. In the vacuum-packed samples, growth started after about 8 days, while in MA packing the growth did not start until after roughly 24 days of storage at 8 °C. This indicates growth inhibition caused by CO2 (Figure 6).
FIGURE 6. Mince inoculated with a cocktail of B. cereus spores and stuffed in casings. Notes: Mince in casings heated to F70 °C = 20 minutes, chilled, cut into slices, and MA or vacuum packed in packaging material with OTR 50 ml/m2 (storage temperature of 8 °C, pH of 6.3, 3 percent salt in aqueous phase, 0.6 percent natural L-lactate/water).
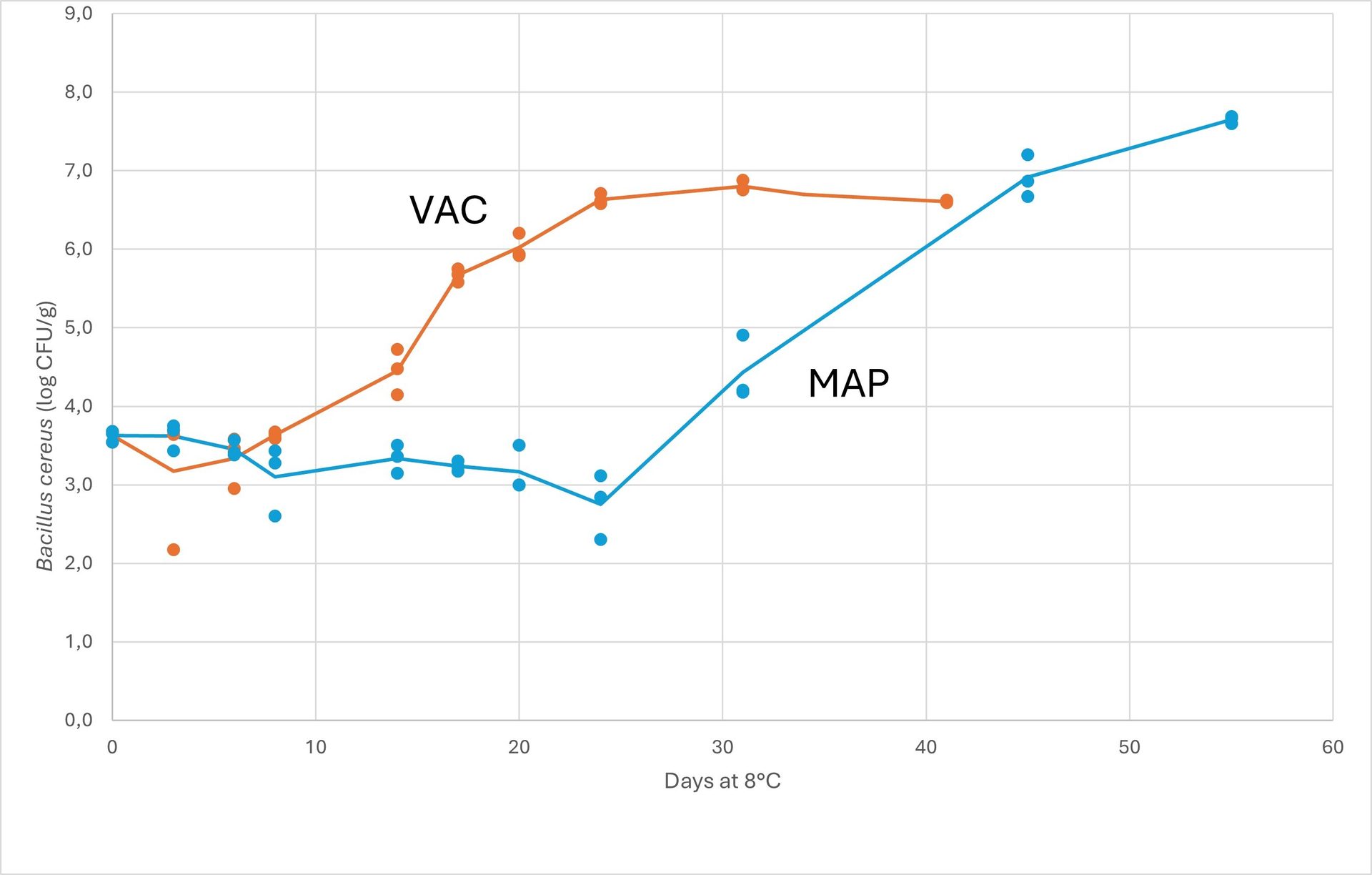
Temperature and Preservation
If the temperature is kept low, it does not take much preservation to prevent the growth of B. cereus in vacuum- and MA-packaged meat products. Figure 7 and Figure 8 show the effect of preservation in vacuum-packed deli meat stored at 10 °C and 5 °C, respectively. If kept at an unfavorably elevated temperature (10 °C, see Figure 7), B. cereus grows readily in vacuum-packed products with added 1 percent or 2 percent NaCl (about 1.7 percent or 3 percent SAP). Maximum numbers were reached after 12 days. Adding 1.5 percent Na-lactate only slightly reduced the growth, but the addition of 3 percent Na-lactate completely inhibited growth for 90 days. In most samples, a decline in culturable B. cereus was observed with the addition of 3 percent Na-lactate. Na-nitrite also showed an efficient growth inhibition (Recipe 2.2). Surprisingly, however, the effect was restricted to only approximately 40 days. After that, growth occurred and maximum numbers were reached at day 90 (4.5 log growth in 50 days). This shows the importance of long-term studies in challenge tests.
FIGURE 7. Growth of B. cereus in vacuum-packed deli meat stored at 10 °C. Notes: Mince inoculated with a cocktail of B. cereus spores, stuffed in casings, heated to F70°C = 24–49 minutes, chilled, cut into slices, vacuum packed in packaging material with OTR 50 ml/m2/24 hours. Recipes: 2.1: pH 6.3, 2.1 percent NaCl, 68 percent water; 2.2: pH 6.2, 2.2 percent NaCl, 67 percent water, 150 ppm nitrite; 3.1: pH 6.2; 1.1 percent NaCl, 65 percent water; 3.2: pH 6.2, 1.1 percent NaCl, 66 percent water, 1.5 percent Na-lactate; 3.3 pH 6.2, 1.1 percent NaCl, 66 percent water, 3 percent Na-lactate. All recipes: approximately 0.6 percent natural L-lactate/water.
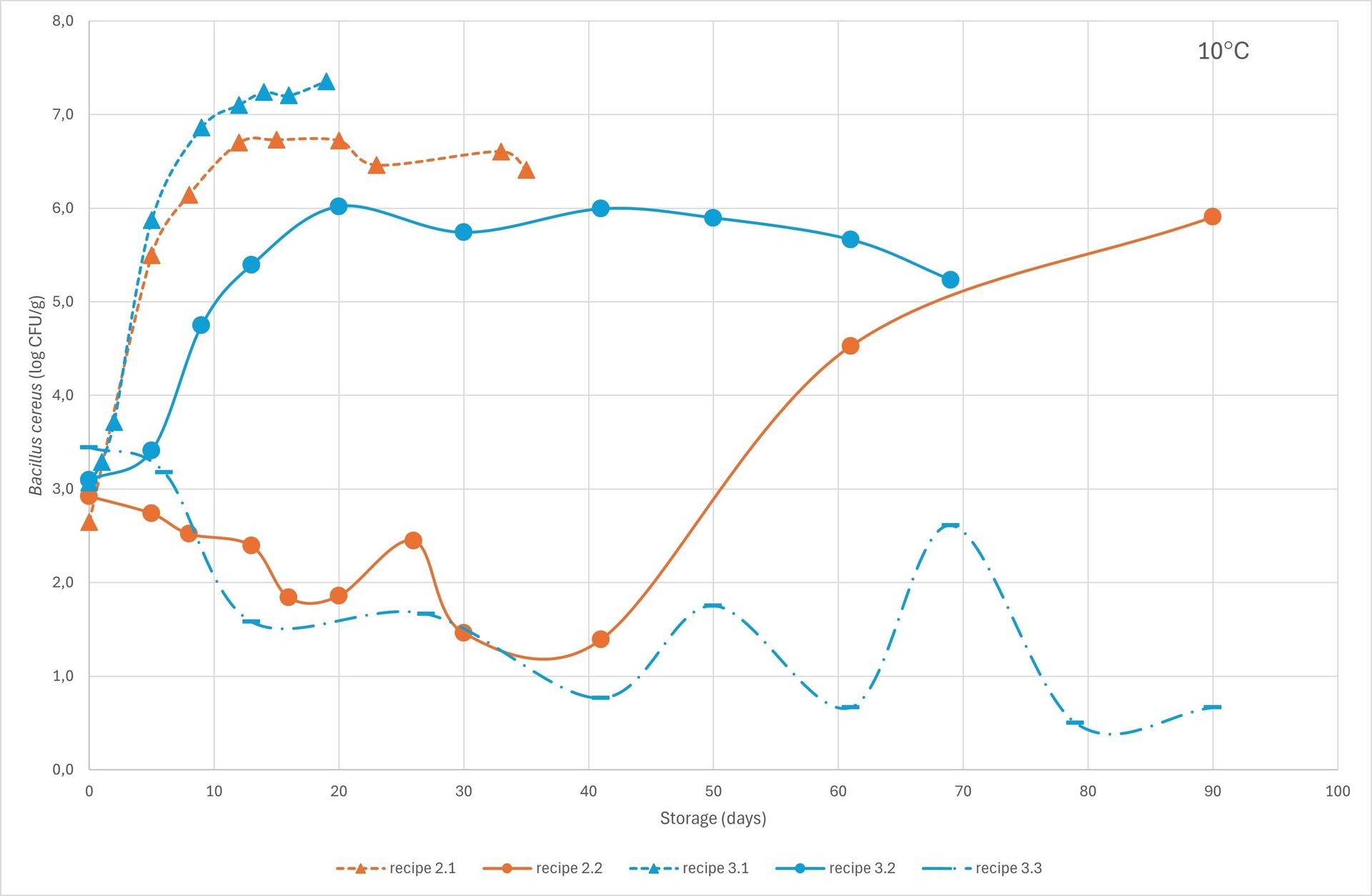
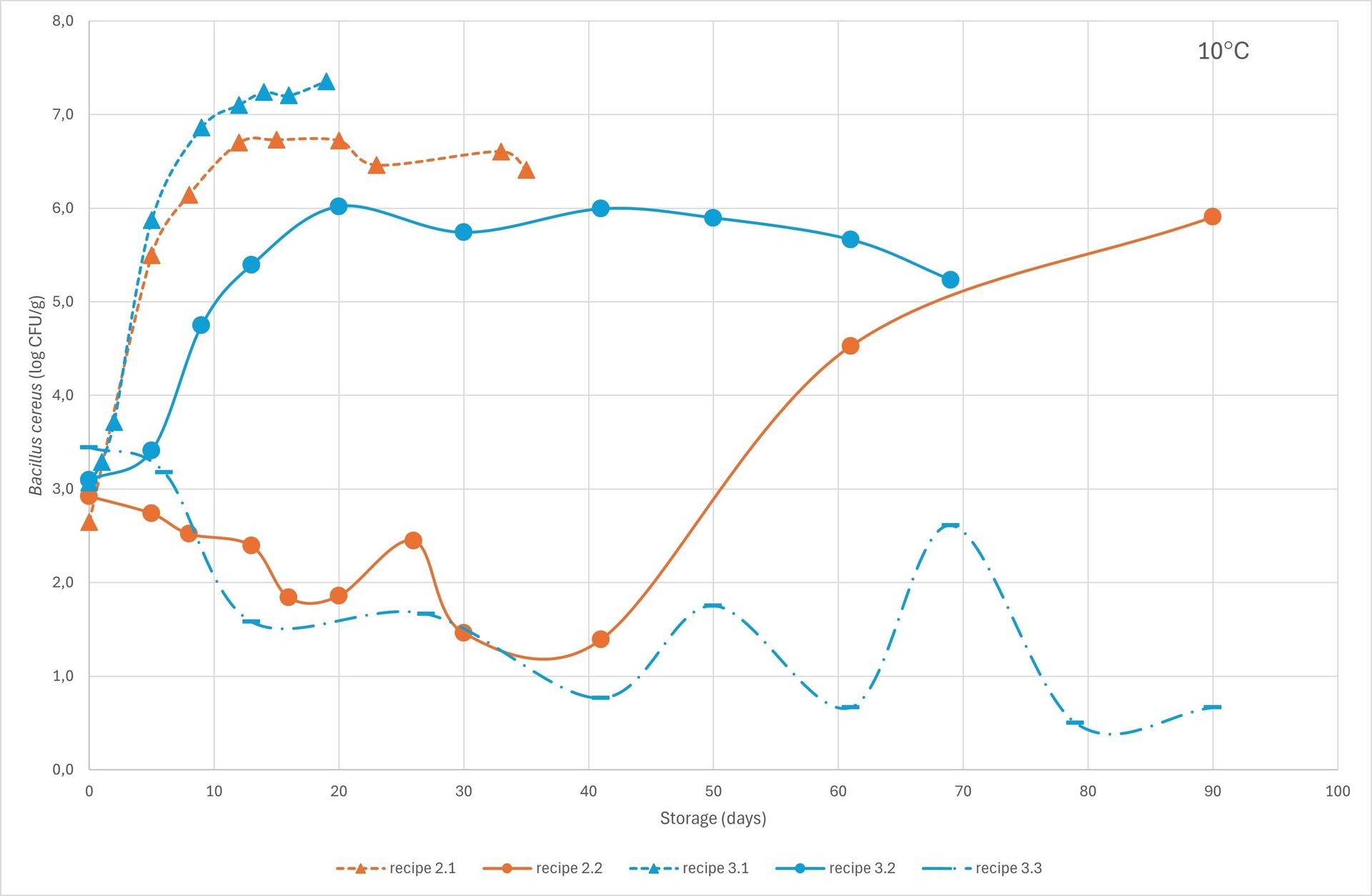
When stored at a lower temperature (5 °C, see Figure 8), the growth of B. cereus was restricted compared to 10 °C. For example, the maximum number of bacteria reached in Recipe 2.1 (2.1 percent NaCl added) and 3.1 (1.1 percent NaCl added) was 2-log lower at 5 °C than at 10 °C (4–5 log at 5 °C vs. 6.5–7.5 log at 10 °C). If the product with added 1.1 percent NaCl received an additional 1.5 percent Na-lactate (Recipe 3.2), then no growth was observed; in fact, there was a reduction of B. cereus. Similarly, 150 ppm Na-nitrite (Recipe 2.2) inhibited growth with a slow reduction in culturable B. cereus during 90 days of storage at 5 °C.
FIGURE 8. Growth of B. cereus in vacuum-packed deli-meat stored at 5 °C. Notes: Mince inoculated with a cocktail of B. cereus spores, stuffed in casings, heated to F70°C = 24–49 minutes, chilled, cut into slices, vacuum packed in packaging material with OTR 50 ml/m2/24 hours. Recipes: 2.1: pH 6.3, 2.1 percent NaCl, 68 percent water; 2.2: pH 6.2, 2.2 percent NaCl, 67 percent water, 150 ppm nitrite; 3.1: pH 6.2; 1.1 percent NaCl, 65 percent water; 3.2: pH 6.2, 1.1 percent NaCl, 66 percent water, 1.5 percent Na-lactate. All recipes: approximately 0.6 percent natural L-lactate/water.
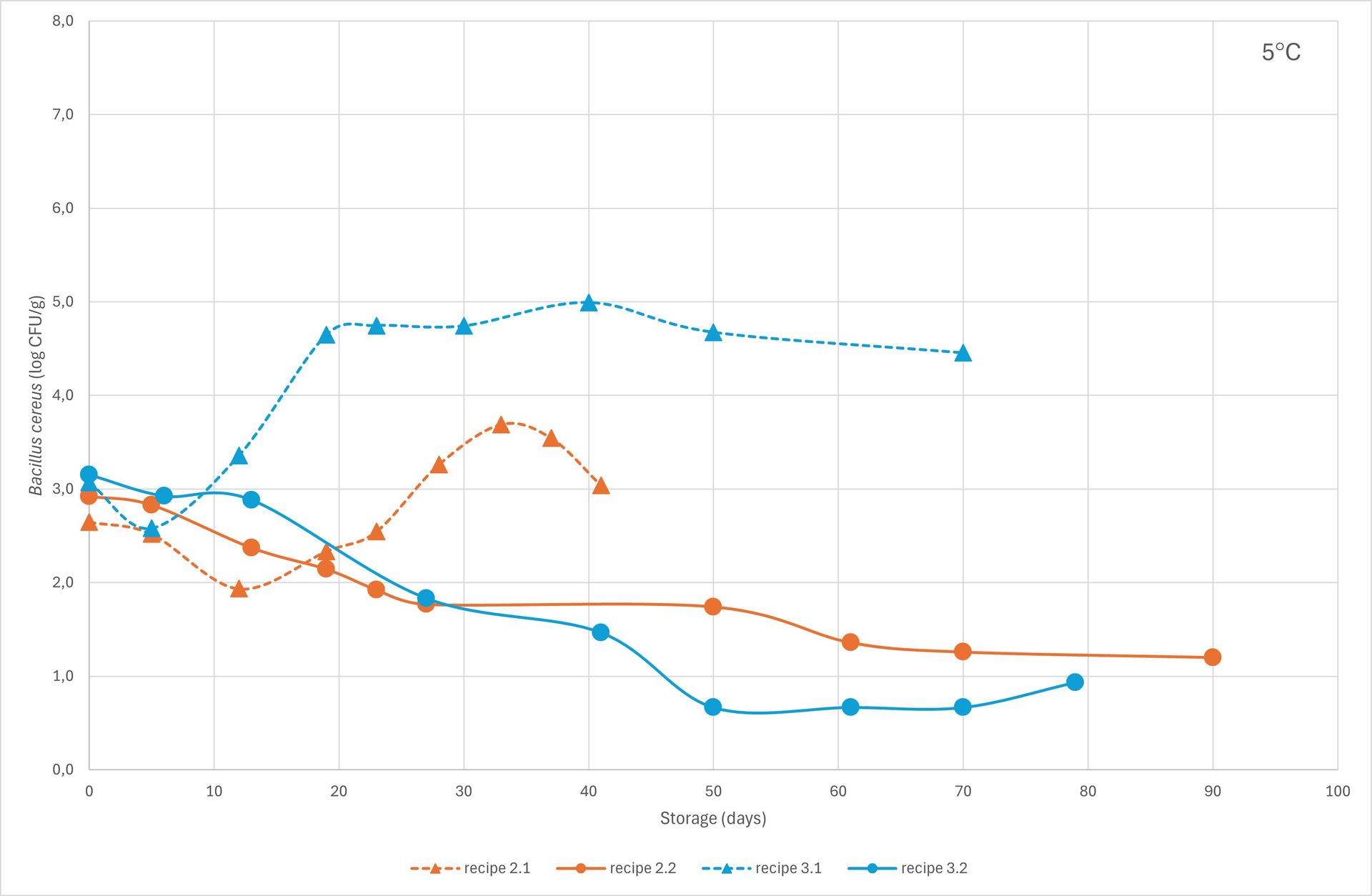
The effect of Na-nitrite and Na-lactate is interesting in relation to the ability to produce ready-to-eat meals with a low sodium content and a long shelf life in connection with sous vide production. All of these findings are crucial to producing safe meat products, especially considering the trend toward adding more vegetables and other plant-based ingredients to deli meats. B. cereus is more often found in plant-based products than in meat, which calls for paying close attention to the way these products are preserved. Another challenge with mixing plant-based products and meat (e.g., in sausages) is that pH, in some cases, may increase.
Model Development
Based on these findings and ongoing experiments, DMRI is in the process of developing a mathematical model for predicting growth of B. cereus in sliced deli meats with or without the addition of plant-based ingredients. This is done through a number of challenge tests, where meat mince (with or without added plant-based ingredients) is inoculated with a cocktail of B. cereus spores, heat treated, and vacuum or MA packaged (30 percent CO2/70 percent N2) with an oxygen residue of about 0.5 percent. Various preservatives are added to the products, which are then stored at 5–10 °C (Table 2).
TABLE 2. Overview of parameters and ranges included in the B. cereus model
The model is expected to be available on DMRI Predict in early 2026, thereby enabling producers to predict growth with the variables and ranges listed in Table 2.
Takeaway
Using predictive models for shelf life and food safety is a strategic choice that offers substantial advantages to the food industry. Predictive models enhance food safety by identifying and mitigating potential spoilage and contamination risks, thereby reducing the incidence of foodborne illnesses. Furthermore, they provide good shelf life estimates, which enable more effective inventory management and significantly minimize food waste and associated costs.
In addition to reducing waste, predictive models are cost-efficient as they diminish the need for extensive laboratory testing, conserving both time and resources while optimizing production and storage processes. They also play a crucial role in regulatory compliance by providing data-driven insights that help ensure adherence to food safety standards.
Moreover, predictive models contribute to sustainability by reducing waste and optimizing the use of resources, promoting more environmentally friendly food production practices. In summary, these models enhance safety, efficiency, and sustainability, making them invaluable tools for the modern food industry.
Acknowledgments
The work to develop and update DMRI Predict has been funded with financial support from the Danish Pig Levy Fund and the Danish Agency for Higher Education and Science.
References
- Danish Technological Institute (DTI). Danish Meat Research Institute (DMRI). "DMRI Predict." 2024. https://dmripredict.dk.
- McMillin, K.W. "Chapter: Modified Atmosphere Packaging." In: Demirci, A., H. Feng, and K. Krishnamurthy (Eds.). Food Safety Engineering. Springer, Cham (May 2020): 693–718. https://doi.org/10.1007/978-3-030-42660-6_26.
- Taylor, T.M., S. Ravishankar, K. Bhargava, and V.K. Juneja. "Chapter: Chemical Preservatives and Natural Food Antimicrobials." In: Doyle, M.P., F. Diez-Gonzalez, and C. Hill (Eds.). Food Microbiology: Fundamentals and Frontiers. American Society for Microbiology. ASM Press (May 2019): 705–731.
- Björkroth, K.J., P. Vandamme, and H.J. Korkeala. "Identification and Characterization of Leuconostoc carnosum, Associated with Production and Spoilage of Vacuum-Packaged, Sliced, Cooked Ham." Applied and Environmental Microbiology 64, no. 9 (September 1998): 3313–3319. https://doi.org/10.1128/AEM.64.9.3313-3319.1998.
- Mejlholm, O. and P. Dalgaard, P. "Development and validation of an extensive growth and growth boundary model for psychrotolerant Lactobacillus spp. in seafood and meat products." International Journal of Food Microbiology 167, no. 2 (October 2013): 244–260. https://doi.org/10.1016/j.ijfoodmicro.2013.09.013.
- Bautista, D.A. "Chapter: Spoilage Problems: Problems Caused by Bacteria." In: Batt, C.A. and M.L. Tortorello (Eds.). Encyclopedia of Food Microbiology (2nd Ed.). Elsevier, 2014: 465–470. https://doi.org/10.1016/B978-0-12-384730-0.00314-1.
- Lonvaud-Funel, A. "Chapter: Leuconostocaceae Family." In: Batt, C.A. and M.L. Tortorello (Eds.). Encyclopedia of Food Microbiology (2nd Ed.). Elsevier, 2014: 455-465. https://doi.org/10.1016/B978-0-12-384730-0.00185-3.
- Raimondi, S., G. Spampinato, F. Candeliere, A. Amaretti, P. Brun, I. Castagliuolo, and M. Rossi. "Phenotypic Traits and Immunomodulatory Properties of Leuconostoc carnosum Isolated from Meat Products." Frontiers in Microbiology 12 (2021): 1–12. https://doi.org/10.3389/fmicb.2021.730827.
- Samelis, J. and A. Kakouri. "Growth Inhibitory and Selective Pressure Effects of Sodium Diacetate on the Spoilage Microbiota of Frankfurters Stored at 4 °C and 12 °C in Vacuum." Foods 10, no. 1 (2021): 1–20. https://doi.org/10.3390/foods10010074.
- U.S. Department of Agriculture (USDA). "ComBase Predictive Models." http://www.combase.cc.
- Ji, L.-l., Y. Wei, D. He, X.-l. Chen, T.-t. Fu, Y. Zhang, and L.-m. Zhao. "Abstract: Study on acid production characteristics of three strains of lactic acid bacteria isolated and purified from fermented sausage." China Condiment 45, no. 2 (2020): 36–39. https://doi.org/10.3969/j.issn.1000-9973.2020.02.009.
- U.S. Food and Drug Administration (FDA). "Bacillus cereus and other Bacillus species." In: Bad Bug Book: Foodborne Pathogenic Microorganisms and Natural Toxins, 2nd Ed. (2012): 92–95.
- Thorsen, L., B.B. Budde, A.G. Koch, and T.D. Klingberg. "Effect of modified atmosphere and temperature abuse on the growth from spores and cereulide production of Bacillus weihenstephanensis in a cooked chilled meat sausage." International Journal of Food Microbiology 130, no. 3 (2009): 172–178. https://pubmed.ncbi.nlm.nih.gov/19230998/.
Anette Granly Koch, PhD. Is the Scientific Manager for the Danish Meat Research Institute (DMRI) at the Danish Technological Institute (DTI).
Gry Carl Terrell, M.Sc. is the Business Manager for DMRI at the DTI.